Pioneering the Way: Rare Disease Trials with Machine Learning
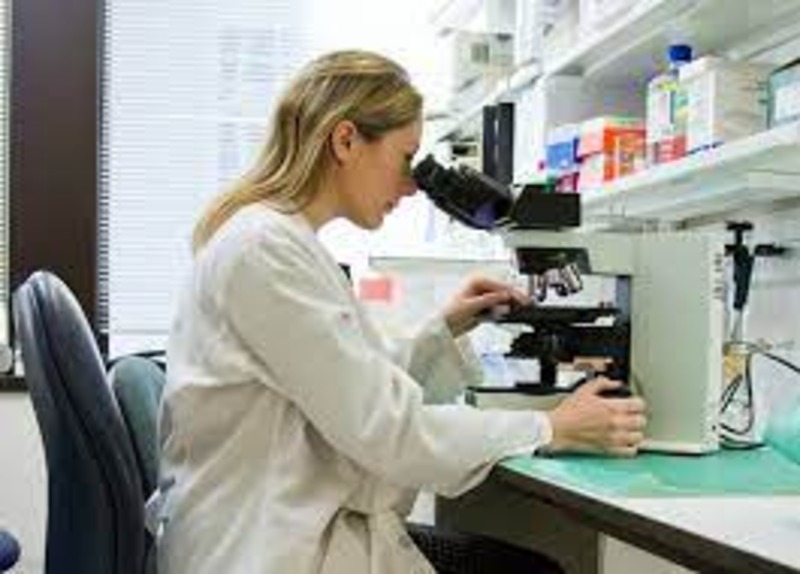
Clinical Research Course, Clinical Research Training, and the Clinical Research Training Institute offer the Best Clinical Research Course and Top Clinical Research Training. But what happens when you combine their expertise with the power of Machine Learning (ML) to tackle one of the most challenging areas of clinical research – rare disease trials?
The Unique Challenges of Rare Disease Trials
Rare diseases are, by definition, uncommon. This rarity makes finding and enrolling patients for clinical trials incredibly challenging. For pharmaceutical companies, researchers, and patients, this poses a significant hurdle.
Machine Learning’s Entrance into Rare Disease Trials
Machine Learning, a subset of artificial intelligence, has begun to transform the landscape of rare disease trials in remarkable ways:
-
Patient Identification: ML algorithms can analyze vast datasets, identifying potential trial candidates by sifting through electronic health records, genetic databases, and clinical notes.
-
Trial Design: ML can optimize trial design by considering variables such as patient demographics, disease progression, and response to treatment.
-
Data Analysis: Rare disease trials generate limited data, making every data point precious. ML techniques can maximize the utility of available data and extract valuable insights.
-
Real-Time Monitoring: ML enables real-time monitoring of patients in remote locations, offering continuous data collection and analysis, which is especially beneficial for diseases that require ongoing observation.
The Role of Clinical Research Training
For clinical research professionals to fully harness the potential of ML in rare disease trials, proper training is essential. Clinical Research Course, Clinical Research Training, and the Clinical Research Training Institute are instrumental in providing the Best Clinical Research Course and Top Clinical Research Training. Here’s how they contribute:
-
Data Management Skills: Clinical research professionals need the skills to manage and prepare data for ML algorithms. This involves cleaning and transforming data, a crucial step for accurate analysis.
-
Ethical Considerations: Training emphasizes ethical data usage, ensuring patient privacy and compliance with regulations.
-
Interdisciplinary Approach: Rare disease trials often require collaboration among professionals from various disciplines, and the training equips individuals to work cohesively in these interdisciplinary teams.
-
Adaptive Learning: As the field of ML in clinical research evolves, so do the training programs. They adapt to new tools and techniques, ensuring that professionals stay up-to-date.
Case Studies in Rare Disease Trials
To illustrate the impact of ML in rare disease trials, let’s consider a few real-world examples:
-
Pompe Disease: This rare genetic disorder leads to muscle weakness and affects both adults and children. ML algorithms have helped identify potential patients more swiftly and design more effective trials.
-
Cystic Fibrosis: ML has improved the patient screening process, allowing for the selection of individuals with specific genetic profiles for personalized therapies.
-
Huntington’s Disease: ML is aiding in the analysis of patient data to identify patterns and markers that can guide treatment strategies.
The Future of Rare Disease Trials with ML
The potential of Machine Learning in rare disease trials is vast:
-
Early Diagnosis: ML can enable early diagnosis of rare diseases, giving patients a better chance of receiving timely treatment.
-
Personalized Therapies: ML algorithms can help in the development of personalized therapies tailored to a patient’s unique genetic makeup and disease characteristics.
-
Expanded Access: Machine Learning can expand access to rare disease trials by identifying potential participants globally, increasing the chances of successful trial completion.
-
Real-World Data: ML can utilize real-world data to assess treatment outcomes, providing valuable insights that complement traditional clinical trial data.
In Conclusion
Rare disease trials have historically faced numerous obstacles, from patient identification to data analysis. The integration of Machine Learning, along with the knowledge and expertise provided by Clinical Research Course, Clinical Research Training, and the Clinical Research Training Institute in the Best Clinical Research Course and Top Clinical Research Training, is revolutionizing the field. With the potential for early diagnosis, personalized treatments, and expanded access to trials, ML is making rare disease research not so rare anymore. As it continues to evolve, ML promises to play a pivotal role in improving the lives of individuals with rare diseases and advancing the field of clinical research as a whole.